Unified data means faster AI: Here’s how to unleash its potential
Harnessing generative AI’s revolutionary potential requires enterprises to take a revolutionary approach to organizing IT assets.
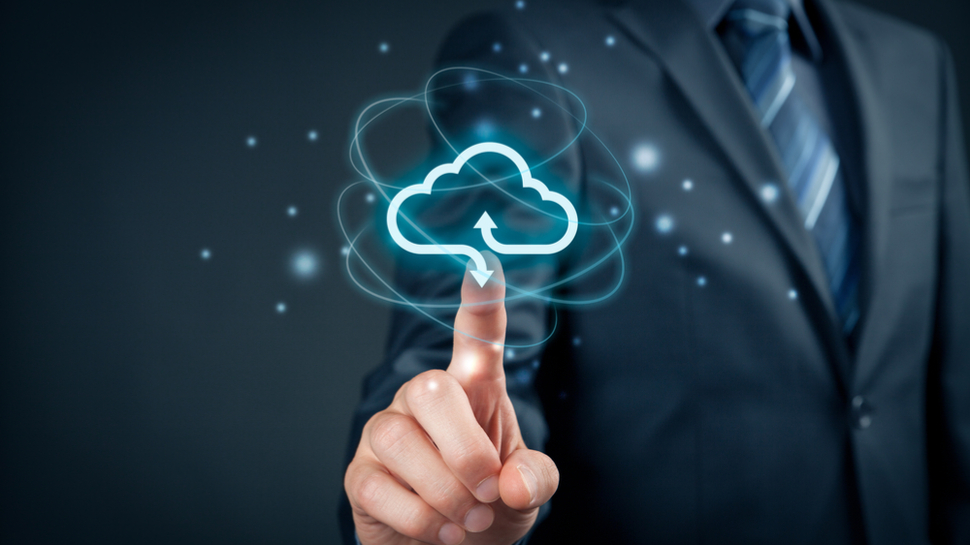
Over the past two years, we have seen AI dominate the global conversation as a possible solution to address massive shifts in people, data, and work. Today, generative AI models can absorb and synthesize many types of data – from video to code and even molecular structures. Making strategic investments in this emerging technology has the potential to help businesses offer a competitive advantage, uncover new business opportunities, and address key challenges across critical functions like customer service, supply chains, and more sustainable operations.
However, for AI to be successful, you must be able to access an enormous amount of data that you can trust. The more data there is – and the more diverse it is – the more accurate your algorithms used to train AI for specific business needs will be. But, for many organizations, the data required for training may not be available in a unified way. Data tends to reside all over the place, in siloed on-prem data centers and spread across public and private clouds often administered by different parts of the business that don’t communicate. Finding the assets you need in such a complex IT environment can often feel like playing a game of hide-and-seek, making it extremely difficult to train, tune, and leverage AI.
As this technology continues to advance, enterprises are at an inflection point. A recent study from the IBM Institute for Business Value shows that 60% of organizations are not yet developing a consistent, enterprise-wide approach to generative AI. To realize AI’s full potential and speed the pace of innovation for specific business needs, I believe that enterprises must first rethink their infrastructure landscape. First, understand where your critical data and applications are and how those locations will leverage AI. Then equip those strategic locations with secured hardware and appropriate high-performance capabilities (accelerators and high-performance storage). Use a consistent set of platform technologies – data management, AI, observability, security, etc. across each of these locations, to speed time to AI value. This platform approach is what we at IBM call hybrid by design.
Today’s default barriers
One of the main roadblocks enterprises face when it comes to realizing the full transformative power of AI is their IT environment. Historically, AI experiments have largely occurred in isolation from one another, with different business divisions pursuing their own separate priorities. For example, a business’ marketing arm might use AI to develop customer segmentation and generate personalized offers. At the same time, the supply chain team could be running AI on a different set of data to improve its management processes. With no top-down, uniform approach to innovation, these efforts will often result in fragmented tech stacks, with data relegated to different clouds with disparate formats and protocols. This is what we call hybrid by default.
While some of these isolated experiments can yield promising results, if they are each implemented independently, their individual overheads can pile up as budgetary dead weight known as tech debt. Even if many of the disjointed AI processes are successful, the collective tech environment will still grow increasingly bloated, running up costs and hampering the agility to innovate. This default progression, where data becomes duplicated and sprawled across a disunified “Frankencloud” environment, prevents enterprises from deriving the kind of game-changing insights that can come from a holistic view of their wealth of data. Worse yet, the lack of cohesion can also make efforts to secure sensitive data against breaches far more complicated and costly.
An intentional data and platform plan, on the other hand, can alleviate these burdens and open the door to countless competitive advantages. With a coordinated data lake, catalog, and governance strategy, implemented based on hybrid cloud architecture, businesses can use AI and customize Large Language Models (LLMs) for a wide range of use cases spanning the gamut of business functions. Consider the above example of experimentation: Rather than being limited to separate insights on customers and supply chains, the business could apply AI across both sets of data to predict sales trends and adjust inventory levels, preventing stockouts and overstocks, reducing costs, and improving customer satisfaction.
This is just the beginning of what’s possible with a hybrid-by-design architecture.
Getting out of “debt”
Scaling AI to enable these insights requires a foundation of unified hardware and cloud-based solutions. While this can be a major undertaking, continually modernizing IT infrastructure is essential for allowing AI to work as it is expected to, as well as for maintaining data governance and security. If these changes are not made, enterprises are at risk of incurring or increasing tech debt. Additionally, adopting AI before this debt is “paid” can result in less effective AI and more distributed data.
When executed properly, a hybrid-by-design approach aims to eliminate the challenges enterprises may face as they move to AI, including skills, costs and security concerns. Let’s take technical debt for example. With a hybrid by design approach, organizations can make it possible for every business unit to share the same data, applications, and policies between on-premises infrastructure, private and public clouds, and the edge—minimizing budgetary waste and enabling rapid scalability by tuning and deploying AI wherever it resides. However, hybrid by design is more than just modernizing existing technology. It is a gradual approach that preserves existing resources and streamlines priorities.
Collaboration across the C-suite – the CIO, CAIO, CISO, and CRO having conversations early in the journey will enable optimization of implementation cost, data protection, and business outcomes for AI. Businesses should start by focusing on a few high-leverage use cases, then standardize implementation using common data, AI, and management platform elements. With tight prioritization, enterprises have the ability to both deliver frontloaded returns and avoid diluting their resources chasing disparate initiatives—in essence, the behavior that caused their hybrid by default tech debt in the first place.
A design for the future
Investment in generative AI is expected to grow nearly four-times over the next two to three years, according to research from the IBV Institute for Business Value, yet it remains a fraction of total AI spend. To harness generative AI’s revolutionary potential, enterprises need to take a revolutionary approach to organizing their IT assets.
A well-designed hybrid cloud architecture can afford enterprises the agility to train, modify, and integrate these new AI capabilities into their workflows at the scale necessary for true business transformation. The possibilities are vast: solutions like advanced automation, real-time analysis, and 24/7 customer engagement can lower operating costs, increase revenue, and lay the foundation for future gains.
We've featured the best AI tools currently available.
This article was produced as part of TechRadarPro's Expert Insights channel where we feature the best and brightest minds in the technology industry today. The views expressed here are those of the author and are not necessarily those of TechRadarPro or Future plc. If you are interested in contributing find out more here: https://www.techradar.com/news/submit-your-story-to-techradar-pro
What's Your Reaction?
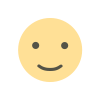
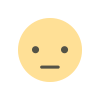
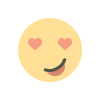
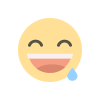
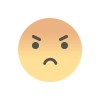
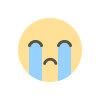
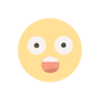