How to Build AI That Customers Can Trust
Trust and transparency in AI have undoubtedly become critical to doing business. As AI-related threats escalate, security leaders are increasingly faced with the urgent task of protecting their organizations from external attacks while establishing responsible practices for internal AI usage. Vanta’s 2024 State of Trust Report recently illustrated this growing urgency, revealing an alarming rise […] The post How to Build AI That Customers Can Trust appeared first on Unite.AI.
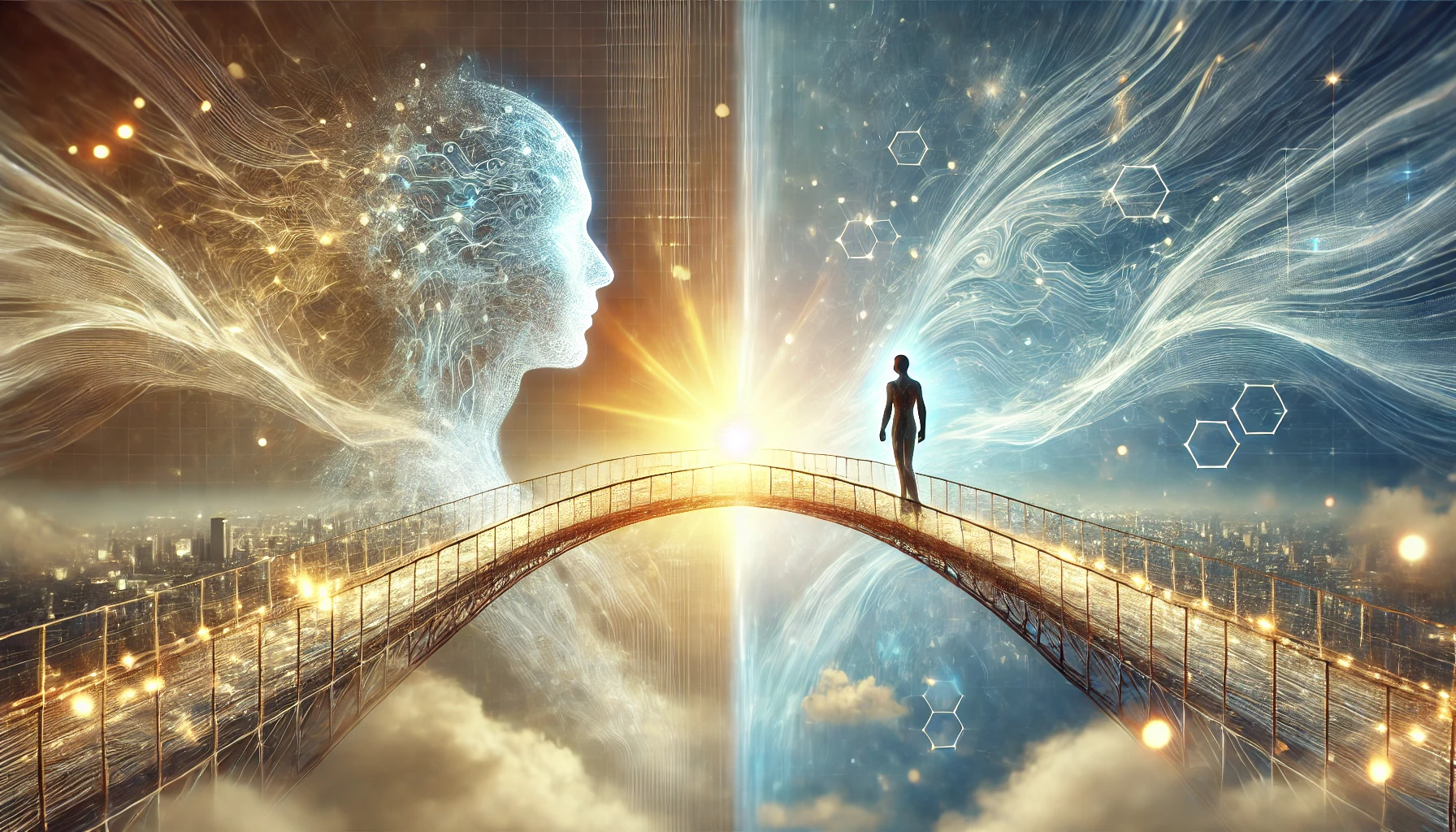

Trust and transparency in AI have undoubtedly become critical to doing business. As AI-related threats escalate, security leaders are increasingly faced with the urgent task of protecting their organizations from external attacks while establishing responsible practices for internal AI usage.
Vanta’s 2024 State of Trust Report recently illustrated this growing urgency, revealing an alarming rise in AI-driven malware attacks and identity fraud. Despite the risks posed by AI, only 40% of organizations conduct regular AI risk assessments, and just 36% have formal AI policies.
AI security hygiene aside, establishing transparency on an organization's use of AI is rising to the top as a priority for business leaders. And it makes sense. Companies that prioritize accountability and openness in general are better positioned for long-term success.
Transparency = Good Business
AI systems operate using vast datasets, intricate models, and algorithms that often lack visibility into their inner workings. This opacity can lead to outcomes that are difficult to explain, defend, or challenge—raising concerns around bias, fairness, and accountability. For businesses and public institutions relying on AI for decision-making, this lack of transparency can erode stakeholder confidence, introduce operational risks, and amplify regulatory scrutiny.
Transparency is non-negotiable because it:
- Builds Trust: When people understand how AI makes decisions, they’re more likely to trust and embrace it.
- Improves Accountability: Clear documentation of the data, algorithms, and decision-making process helps organizations spot and fix mistakes or biases.
- Ensures Compliance: In industries with strict regulations, transparency is a must for explaining AI decisions and staying compliant.
- Helps Users Understand: Transparency makes AI easier to work with. When users can see how it works, they can confidently interpret and act on its results.
All of this amounts to the fact that transparency is good for business. Case in point: research from Gartner recently indicated that by 2026, organizations embracing AI transparency can expect a 50% increase in adoption rates and improved business outcomes. Findings from MIT Sloan Management Review also showed that companies focusing on AI transparency outperform their peers by 32% in customer satisfaction.
Creating a Blueprint for Transparency
At its core, AI transparency is about creating clarity and trust by showing how and why AI makes decisions. It’s about breaking down complex processes so that anyone, from a data scientist to a frontline worker, can understand what’s going on under the hood. Transparency ensures AI is not a black box but a tool people can rely on confidently. Let’s explore the key pillars that make AI more explainable, approachable, and accountable.
- Prioritize Risk Assessment: Before launching any AI project, take a step back and identify the potential risks for your organization and your customers. Proactively address these risks from the start to avoid unintended consequences down the line. For instance, a bank building an AI-driven credit scoring system should bake in safeguards to detect and prevent bias, ensuring fair and equitable outcomes for all applicants.
- Build Security and Privacy from the Ground Up: Security and privacy need to be priorities from day one. Use techniques like federated learning or differential privacy to protect sensitive data. And as AI systems evolve, make sure these protections evolve, too. For example, if a healthcare provider uses AI to analyze patient data, they need airtight privacy measures that keep individual records safe while still delivering valuable insights.
- Control Data Access with Secure Integrations: Be smart about who and what can access your data. Instead of feeding customer data directly into AI models, use secure integrations like APIs and formal Data Processing Agreements (DPAs) to keep things in check. These safeguards ensure your data stays secure and under your control while still giving your AI what it needs to perform.
- Make AI Decisions Transparent and Accountable
Transparency is everything when it comes to trust. Teams should know how AI arrives at its decisions, and they should be able to communicate that clearly to customers and partners. Tools like explainable AI (XAI) and interpretable models can help translate complex outputs into clear, understandable insights. - Keep Customers in Control: Customers deserve to know when AI is being used and how it impacts them. Adopting an informed consent model—where customers can opt in or out of AI features—puts them in the driver’s seat. Easy access to these settings makes people feel in control of their data, building trust and aligning your AI strategy with their expectations.
- Monitor and Audit AI Continuously: AI isn’t a one-and-done project. It needs regular checkups. Conduct frequent risk assessments, audits, and monitoring to ensure your systems stay compliant and effective. Align with industry standards like NIST AI RMF, ISO 42001, or frameworks like the EU AI Act to reinforce reliability and accountability.
- Lead the Way with Internal AI Testing: If you’re going to ask customers to trust your AI, start by trusting it yourself. Use and test your own AI systems internally to catch problems early and make refinements before rolling them out to users. Not only does this demonstrate your commitment to quality, but it also creates a culture of responsible AI development and ongoing improvement.
Trust isn’t built overnight, but transparency is the foundation. By embracing clear, explainable, and accountable AI practices, organizations can create systems that work for everyone—building confidence, reducing risk, and driving better outcomes. When AI is understood, it’s trusted. And when it’s trusted, it becomes an engine for.
The post How to Build AI That Customers Can Trust appeared first on Unite.AI.
What's Your Reaction?
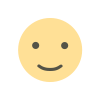
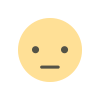
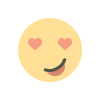
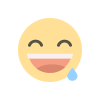
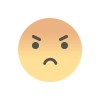
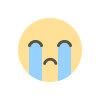
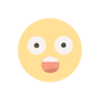