ByteDance Researchers Introduce Tarsier2: A Large Vision-Language Model (LVLM) with 7B Parameters, Designed to Address the Core Challenges of Video Understanding
Video understanding has long presented unique challenges for AI researchers. Unlike static images, videos involve intricate temporal dynamics and spatial-temporal reasoning, making it difficult for models to generate meaningful descriptions or answer context-specific questions. Issues like hallucination, where models fabricate details, further compromise the reliability of existing systems. Despite advancements with models such as GPT-4o […] The post ByteDance Researchers Introduce Tarsier2: A Large Vision-Language Model (LVLM) with 7B Parameters, Designed to Address the Core Challenges of Video Understanding appeared first on MarkTechPost.



Video understanding has long presented unique challenges for AI researchers. Unlike static images, videos involve intricate temporal dynamics and spatial-temporal reasoning, making it difficult for models to generate meaningful descriptions or answer context-specific questions. Issues like hallucination, where models fabricate details, further compromise the reliability of existing systems. Despite advancements with models such as GPT-4o and Gemini-1.5-Pro, achieving human-level video comprehension remains a complex task. Accurate event perception and sequence understanding, coupled with reducing hallucination, are crucial hurdles to overcome.
ByteDance researchers have introduced Tarsier2, a large vision-language model (LVLM) with 7 billion parameters, designed to address the core challenges of video understanding. Tarsier2 excels in generating detailed video descriptions, surpassing models like GPT-4o and Gemini-1.5-Pro. Beyond video descriptions, it demonstrates strong performance in tasks such as question-answering, grounding, and embodied intelligence. With an expanded pre-training dataset of 40 million video-text pairs, fine-grained temporal alignment, and Direct Preference Optimization (DPO) during training, Tarsier2 achieves noteworthy improvements. For example, on the DREAM-1K dataset, it outperforms GPT-4o by 2.8% and Gemini-1.5-Pro by 5.8% in F1 scores.

Technical Innovations and Benefits
Tarsier2 integrates several technical advancements to enhance performance. The model’s architecture includes a vision encoder, vision adaptor, and a large language model, combined in a three-stage training process:
- Pre-training: A dataset of 40 million video-text pairs, enriched with commentary videos that capture both low-level actions and high-level plot details, provides a solid foundation for learning.
- Supervised Fine-Tuning (SFT): Fine-grained temporal alignment during this stage ensures the model accurately associates events with corresponding video frames, reducing hallucination and improving precision.
- Direct Preference Optimization (DPO): This phase employs automatically generated preference data to refine the model’s decision-making and minimize hallucinations.
These advancements not only improve the generation of detailed video descriptions but also enhance the model’s overall versatility across video-centric tasks.
Results and Insights
Tarsier2 achieves impressive results across multiple benchmarks. Human evaluations reveal an 8.6% performance advantage over GPT-4o and a 24.9% improvement over Gemini-1.5-Pro. On the DREAM-1K benchmark, it becomes the first model to exceed a 40% overall recall score, highlighting its ability to detect and describe dynamic actions comprehensively. Furthermore, it sets new performance records on 15 public benchmarks, including tasks like video question-answering and temporal reasoning. In the E.T. Bench-Grounding test, Tarsier2 achieves the highest mean F1 score of 35.5%, underlining its capabilities in temporal understanding. Ablation studies further underscore the critical role of the expanded pre-training dataset and DPO phase in enhancing performance metrics like F1 scores and accuracy.


Conclusion
Tarsier2 marks a significant step forward in video understanding by addressing key challenges such as temporal alignment, hallucination reduction, and data scarcity. ByteDance researchers have delivered a model that not only outperforms leading alternatives in key metrics but also provides a scalable framework for future advancements. As video content continues to dominate digital media, models like Tarsier2 hold immense potential for applications ranging from content creation to intelligent surveillance.
Check out the Paper. All credit for this research goes to the researchers of this project. Also, don’t forget to follow us on Twitter and join our Telegram Channel and LinkedIn Group. Don’t Forget to join our 65k+ ML SubReddit.
What's Your Reaction?
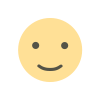
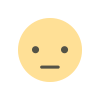
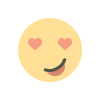
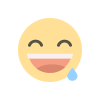
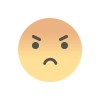
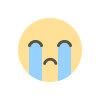
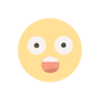